Avoid these 5 mistakes when hiring a data team
- In Big Data, Technology and Insights
- Articles
- 5 min read
Hiring data teams at your attraction can be confusing. Chances are this is a new function for your team which will open a whole new world of understanding of what your organization needs.
1. Unicorn hunting and other expectation gaps
By far the most common pitfall when hiring a data team in a visitor attraction is to go after a unicorn. A data unicorn is a leader who will navigate executive communication and organizational change with ease, be able to drop into the weeds in an instant with the patience to respond to miniscule data integrity errors! They have years of engineering experience in data automation best practice, who can find the time to engage in deep data science research with the skills of a machine learning engineer to operationalize. Sounds great right? But..quite simply: they don’t exist. If you find yourself unicorn hunting, it usually signals a need to reset expectations and make hard decisions on what’s important. Instead, check out our guide on the roles of a data team in visitor attractions.
Another common mistake is overstating a role’s title, usually in order to attract candidates – for example, turning what is really a data analyst position into that of a data scientist, when what you really want is someone to own data integrity, respond to complex queries, inform data automation and facilitate the reporting framework. Overstating titles will misdirect the recruit’s focus and mindset, expectations for others and ultimately set them up to fail – resulting in quick churn when both candidate and colleagues are disappointed. The data role’s location is also important to set expectations – if it is to be embedded in a department like marketing or finance, expect it to be exclusively dedicated to that function and not under expectations to delve into visitor experience or other areas. Ideally, avoid burying the data function in your organizational design and keep it as high as possible, ideally as its own domain.
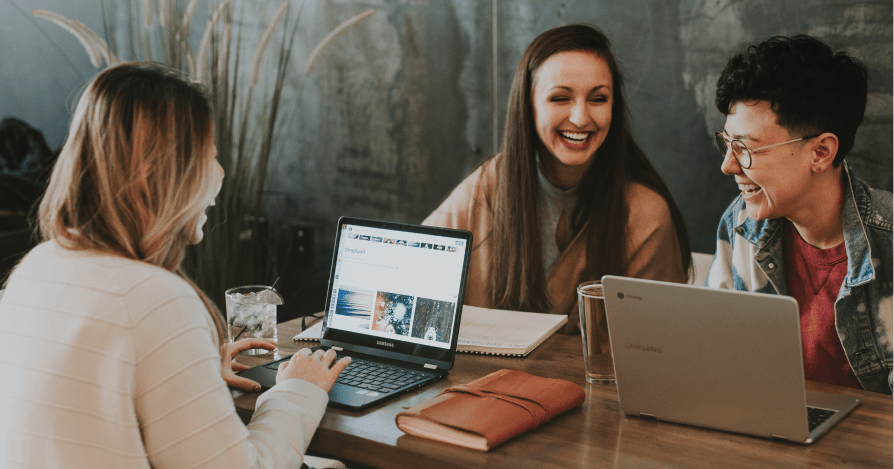
For many cultural institutions, university students or graduates are a popular go to, with cost a heavy factor and data hires notoriously expensive in a competitive labor market. If you are hiring an intern, make sure they have a data knowledgeable lead in house (rather than relying on the student’s academic staff, who won’t have the commercial background or business context needed to guide their work). Give the students small, tightly bound, nice to have problems without dependencies or deadlines on their work – rather than relying on them to deliver for critical problems and core functions of your data agenda.
One last note on this topic: the language of data hires is technically complex, and what seem like similar concepts on candidate applications can mean wildly different things (for example, experience in ‘business analysis’ is not the same thing as ‘business analytics’ – those two letters make a huge difference). Make sure and seek outside expertise if you need support to evaluate technical fit to avoid nasty surprises post hire.
2. Hiring just a builder when you’re going to need a maintenance crew
The total cost of ownership of any custom built technology solution is usually far higher from the support and maintenance than it is from the initial build – even more so for data solutions. So when hiring a data team, it makes sense to hire mostly for this ongoing effort.
When recruiting, this means hiring people who understand, talk to and are not afraid of support and maintenance, because they have a pragmatic reality that in technology, this is most of the job. Candidates that are over enthusiastic about building solutions and neglect to talk to caretaking tend to quickly hop from job to job building things they never stay around to support. For the visitor attraction, this knowledge gap pushes the total cost of ownership even higher with huge risks to the organization, often creating solutions that die with resignations.
This dilemma is particularly true for data science. Given how relatively new the field is, many data scientists’ experience is limited to research, rather than operationalizing models for business use. This may mean both a technical skill gap for which you may need a machine learning engineer to fill and an expectation gap for the candidate on the realities of maintaining operational models – constantly monitoring model integrity, establishing a test harness and conducting continuous improvement experiments – far less glamorous than the next big research project.
3. Not challenging the ‘we can build that’ pitch
The philosophies on the build or buy debate balance total cost of ownership (including the magnitudes of ongoing support and maintenance), lead time and delivery risk, and consider the core business of the visitor attraction. As a general guide, it’s best to ‘buy what’s common, build what’s unique’ – meaning buy solutions which are industry standard for functions you share with other organizations, and save your build investment, energy and risk to funnel into things that make your visitor attraction unique above all others. Despite the pull of curiosity and skill growth in building an inhouse solution, great technical leaders have a balanced and business orientated mindset towards this.
This holds true for the data solution, too. Integrations, data warehouses, dashboards, reports, forecasts and even insights are all common across the industry and best delivered through a productized solution like Dexibit. This will save you needing to hire multiple technical roles such as data engineers and data scientists to manage your core data solution ongoing and dilute knowledge risk. At best, target any build efforts in the data space into going deep on unique custom insights, such as analyzing your collections database, or bespoke digital interactive analytics.
When it comes to data solution evaluation, ensure you have perspectives from a diverse range of end users across departments, seniority and data confidence, rather than a sole technical voice. The needs and user experience demands of data experts versus data consumers vary widely and may even require dual solutions to serve.
A close follow on the buy versus build dilemma is making sure there’s adequate investment into data automation and insight application. At a surface level, a data solution may look simply like hooking up some quick integrations to a visualization tool – however that will result in a big support drag in both obtaining and maintaining data, plus accessing and interpreting it – creating data gatekeepers rather than democratizing data. This inevitably leads to dissatisfied stakeholders, data team boredom from constant processing and ultimately fails to empower the organization, limiting the upside of the data investment.
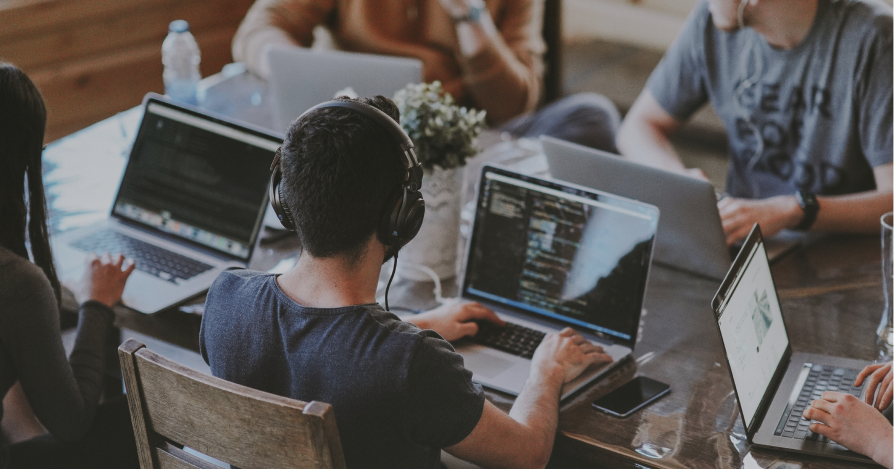
4. Goaling on outputs over outcomes
When working with a data team, give them specific problems to solve. An example could be “upsell revenue is not growing”. Work with the team collaboratively to set goals, such as “increase retail spend by $0.50 per visit by quarter end”. This will drive a focus on results, rapidly iterative action and an experimental mindset. With the addition of quick wins, such as ‘automating a daily retail report’, you’ll quickly turn skeptics into believers.
If your team is instead focused on outputs and deliverables, expect them to spend at least a year forming a data vision and strategy, or extensive audit reports which may never be read, with over glamorized ideas that might take another 3 to 5 years to happen. Data strategies are important, but more effective when they’re informed by validation through hands on, continuous discovery and delivery rather than a big bang approach which is entirely theoretical and speculative.
Another way to heighten focus in your data team is to limit the data sources they have to worry about – in this case for example, they may start simply with visitation numbers and point of sale data. A goal of ‘getting all the data’ will instead send the data team on a never ending mission to integrate everything, at the expense of proving its return, inevitably ending up in the weeds of various data issues with various source systems.
To keep a tight scope, charge your team with getting a basic solution or ‘Minimum Viable Product (MVP)’ operationalized quickly – meaning in the hands of your end users across the organization – then iterate.
5. Over indexing technical expertise over communication and leadership skills
The hardest part of the job for any data role is communication and leadership. Look for candidates who are able to simplify complex ideas for others, bring clarity to confusion and can talk to the lessons they’ve learned in driving data literacy, adoption and usage – things requiring others to change the way they work. These skills are far harder to learn and often more innate than any technical capability and are the difference between seeing outcomes versus frustration.
As a leader, one thing you can do to encourage this is to get your data team out onto the
front line with your visitor services team early and often. Immerse them in your various teams and departments, building relationships across the organization and set the tone for them to take it upon themselves to build their domain knowledge of the visitor attraction.
For more on data best practices at your visitor attraction, download our actionable workbook below:
Workbook: Lead a comprehensive data audit
Data audits don’t have to be hard or expensive. Bring your stakeholder together to discuss their data vision and find passionate champions for informed insight.
To lead an easy and successful data audit, read our latest workbook.
Get insights delivered right to your inbox
Want to learn more about Dexibit?
Talk to one of our expert team about your vision to discover your data strategy and see Dexibit in action.